TECHNOLOGY
ELITE FRAMEWORK
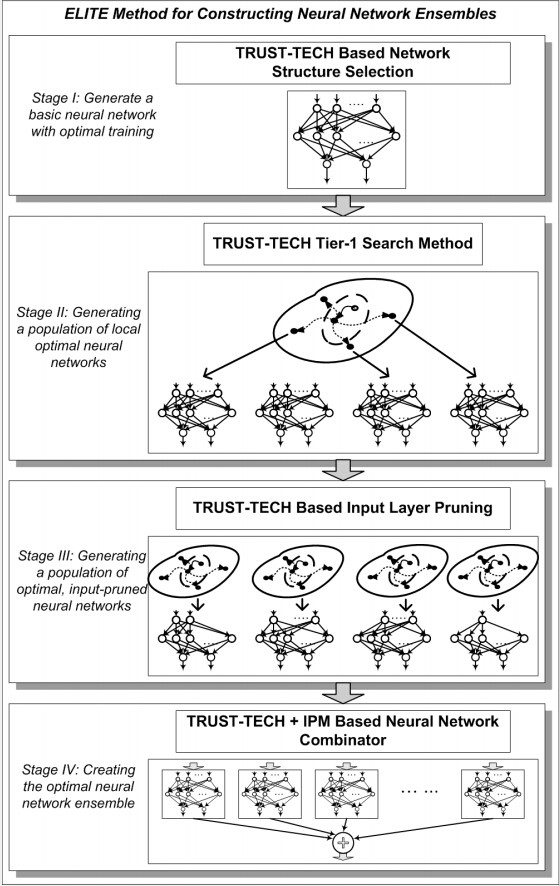
After significant research and development efforts, we at GOT, Inc. have developed an innovative and dynamic framework, termed ELITE for constructing high-quality ensembles of learning models. ELITE is designed to address the two challenging issues in the area of machine learning: the issue of model architecture selection and the issue of optimal model training. There are four stages in ELITE in which a seed learning model is first constructed (Stage I), followed by a family of member learning models (Stage II), each of which is optimally pruned (Stage III), and the optimal ensemble is achieved at Stage IV. Several design tasks in ELITE is formulated as optimization problems and solved by the TRUST-TECH method, which provides a systematic and deterministic way to escape from a local optimal solution and to approach multiple local optimal solutions.
Distinguished features of ELITE include the following:
Diversity: Ensemble members of ELITE are distinct optimal learning models with different optimally-pruned inputs. These members are generated using the proposed saliency based feature selection method. In this manner, diversity of the ensemble members can be achieved.
Accuracy: In ELITE, accuracy of the ensemble members is achieved via selecting high-quality member models from multiple local optimal solutions obtained by the TRUST-TECH based training method.
Optimality: Optimality of the ensemble in ELITE is achieved by optimally combining a set of optimal and pruned member models. Specifically, optimality of the member learning models is attained by using a TRUST-TECH based training method, and by achieving optimality of the combination weights is achieved via solving the associated nonconvex quadratic optimization problem using TRUST-TECH and a local optimizer.